The MATLAB implementation of bilateral filter can be found here or here.
The bilateral filter is a local filter\cite{aurich1995non,smith1997susan,tomasibilateral} that reduces noise in images while preserving edges by means of non-linear combination of local pixel values. However the problem is to find optimal parameter for the bilateral filter in each case of the signal level.
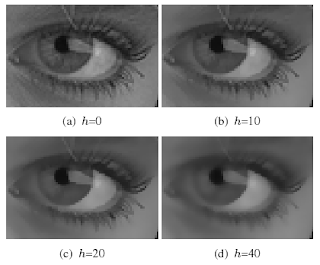
Bilateral filtering using a $5\times5$ square window as $\beta$ and a variable $h$
The bilateral filter replaces a pixel value in an image by a weighted mean of its neighbours considering both their geometric closeness and photometric similarities\cite{bilaterialshotnoise}.
The Gaussian bilateral filter version has a set of parameters that have an important impact on filtering behaviour and performance. The Gaussian bilateral filter is the most used on practice\cite{paris2009fast}:
$v(x) = \frac{1}{C(x)} \sum_{\beta} exp(-\frac{|x-y|^2}{\rho^2}) exp(-\frac{|u(y) - u(x)|^2}{h^2})$
where $\beta$ represents the sliding window, y is a set of 2-D pixel
positions in the sliding window, and x is the 2-D position of
the centred pixel in the sliding window. The u(x) is the intensity
of the pixel at the x position in the original image, v(x) is
the estimated pixel at the x position, $\rho$ and $h$ are the standard deviation of the Gaussian distribution of the geometrical and the intensity weight respectively.
Parameter $\rho$ can be chosen considering the size of the convolution kernel.
Parameter h has to be chosen considering the level of filtering needed for the application.
The paper\cite{bilaterialshotnoise} presents the approach of adaptive parameters choice. But it is more interesting to see the actual noise suppression capabilities of the bilateral filter.
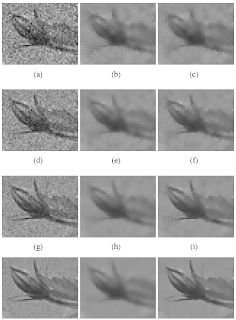
From left to right, the first column shows the noisy images, the second column shows the bilateral filtered images with a h parameter fixed to 100, the third column shows the results obtained with our proposed method.
As stated earlier, the bilateral filter smoothing properties
vary with h. Intuitively we can assume that a low h should be preferable in low noise level, whereas a high h may be necessary in high-noise conditions.
REFERENCES:
\bibitem{bilaterialshotnoise}
H.~Phelippeau, H.~Talbot, M.~Akil, and S.~Bara.
\newblock {Shot noise adaptive bilateral filter}.
\newblock pages 864--867, 2008.
\bibitem{tomasibilateral}
C.~Tomasi and R.~Manduchi.
\newblock {Bilateral filtering for gray and color images}.
\newblock pages 839--846, 1998.
\bibitem{aurich1995non}
V.~Aurich and J.~Weule.
\newblock {Non-linear gaussian filters performing edge preserving diffusion}.
\newblock pages 538--545, 1995.
\bibitem{smith1997susan}
S.M. Smith and J.M. Brady.
\newblock {SUSAN - A new approach to low level image processing}.
\newblock {\em International journal of computer vision}, 23(1):45--78, 1997.
\bibitem{paris2009fast}
S.~Paris and F.~Durand.
\newblock {A fast approximation of the bilateral filter using a signal
processing approach}.
\newblock {\em International journal of computer vision}, 81(1):24--52, 2009.
No comments:
Post a Comment