Introduction
For instance, we have a skewed probability density function and the data generated according to it. Let it be Log-Normal distribution:
$p_{LogNorm}(x;\mu, \sigma) = \frac{1}{x\sqrt{2\pi\sigma^2}}\, e^{-\frac{\left(\ln x-\mu\right)^2}{2\sigma^2}}$
with parameters $\mu = 4.0246$ and $\sigma = 0.0350$ respectively. The data looks like:
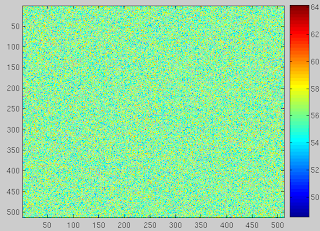
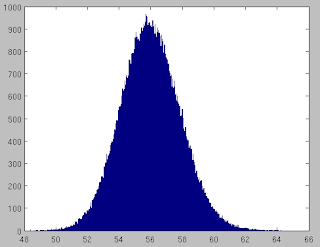
The pdf was numerically estimated using hist function in MATLAB.
The Problem:
I need to make a new pdf that is superimposed by the uniform distribution. The idea is to make a distribution with very long "tail". Well, we can do it accurately, using theoretical expressions of sum of random variables (see, for instance, Probability and random processes by Stark and Woods, Chapter 3). But after all, we need to generate the data using it and not just tinker with it.
The solution
Let's say, we need to add some uniform distribution in order to make the "tail" of summary distribution longer. OK, we can easily generate uniform distribution using rand function in MATLAB or Octave. Generate a uniform distribution of random numbers on a specified interval [a,b]. To do this, multiply the output of rand by (b-a) then add a. Here is MATLAB code:
Isize = 256;Now it is not obvious: if we just add two distributions (either in spatial domain or in Fourier domain using point-wise addition), we will get wrong result:
a=5;
b=50;
I = a + (b-a)*rand(Isize);
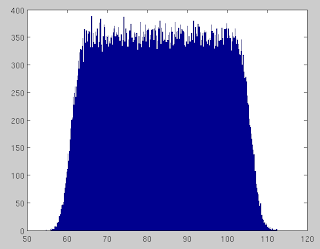
What has happenned? Well, because this is uniform distribution, it just wiped out our gentle Log-Normal data. Hence we need to attenuate the amount of the uniform distribution that we add.
One solution is to add, say, every second data point. Nice idea, but not clever: our data will be periodic that is not desirable. The better solution is to generate another uniform distribuiton [0,1] and make it like a mask for addition of desired data points.
In order to attenuate the influence of the other distribution, we need to add only a few point from the other distribution. Here is the example:
Here our mask will be like:
z = ones(Isize); %% creating a mask of the adding new pdf.
addition_percentage = 0.6;
z = rand(Isize,Isize);
z(z>addition_percentage) = 0;
%%% Erasing the unnecessary points from the other distribution
I = I.*z;
0 0 0.1157 0.2088 0.5074
0.3981 0 0.3826 0.3936 0.1435
0 0.0476 0.5989 0.5155 0.5239
0 0 0 0.4117 0
0 0 0.0858 0 0
0 0.4345 0.4846 0.4592 0
0 0.4591 0.3690 0.1043 0
We can use ceil(z) to make it just 0 or 1, but in our case we want the "tail" to decay. Multiplication of two uniform distribution is not a longer uniform distribution, as one might think :-) It will be triangular-shaped and, if we continue to multiply it more, will tend to be Gaussian, as followed by CLT:
The Central Limit Theorem (CLT) states conditions under which the mean of a sufficiently large number of independent and identically distributed random variables, each with finite mean and variance, will be approximately normally distributed.So then we just add:
I2 = I+Ilong;where I is uniform distribution multiplied by uniform mask (now will be triangular-like), and Ilong is long-tailed distribution. The result pleases the eye, the mind and the soul:
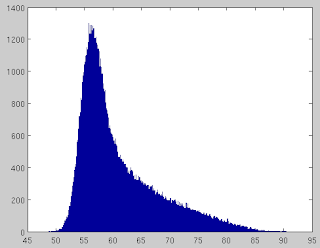
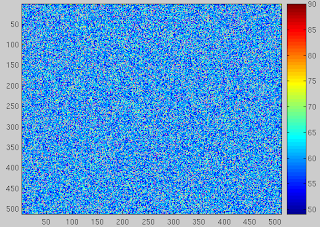
Various thoughts and jots
The sum of two normal distributions is itself a normal distribution:
N(mean1, variance1) + N(mean2, variance2) ~ N(mean1 + mean2, variance1 + variance2)
This is all on wikipedia page.
Be careful that these really are variances and not standard deviations.